For ecommerce SEO, shop category pages have been a key focus. But that could change.
The presence of Google Shopping in the form of Product Grids and as part of AI Overviews is increasing rapidly. This puts Google Shopping as the focus of ecommerce SEO.
Google’s semantic database behind shopping is the Google Shopping Graph, so it’s time you consider shopping graph optimization.
Applications based on generative AI will change many users’ search behavior. Research will become more interactive, individualized, precise and faster.
It will be 2.8 times faster in the future thanks to generative AI, according to Microsoft.
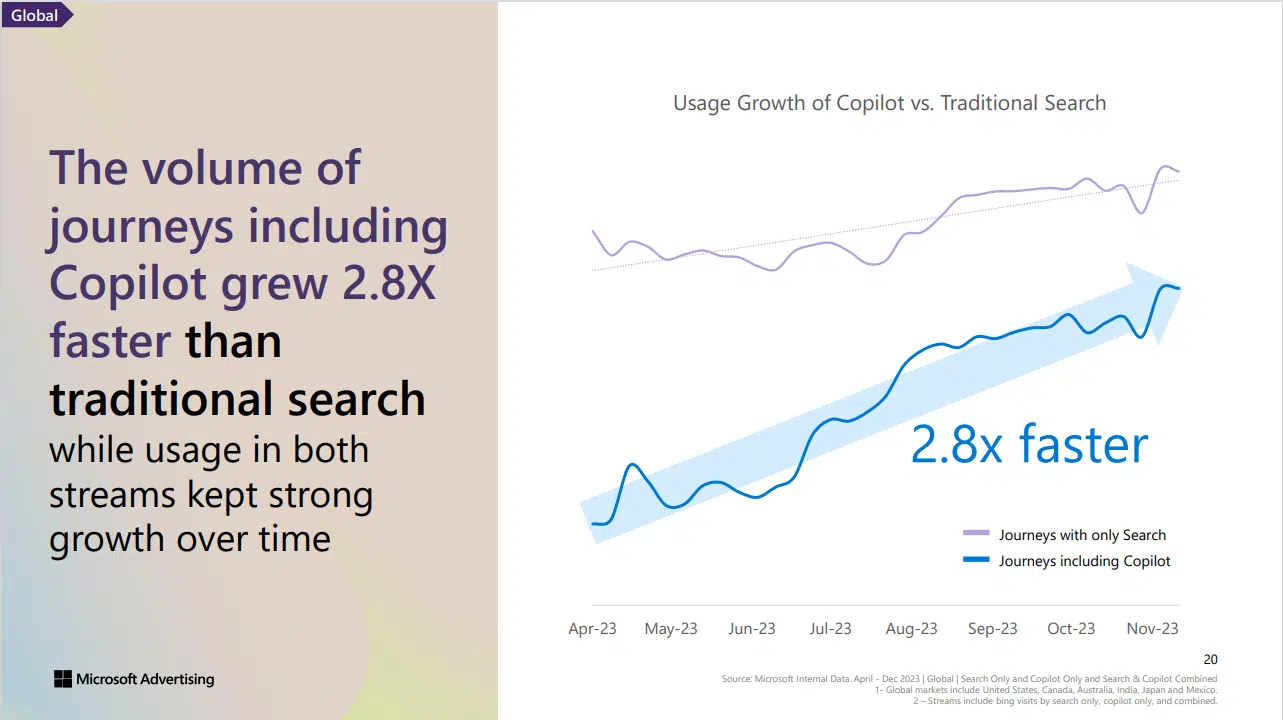
This means that in the future, users will click less on search results and will need significantly fewer touchpoints to find out more about products.
The messy middle is shortened thanks to hybrid research using AI-generated answers and classic search.
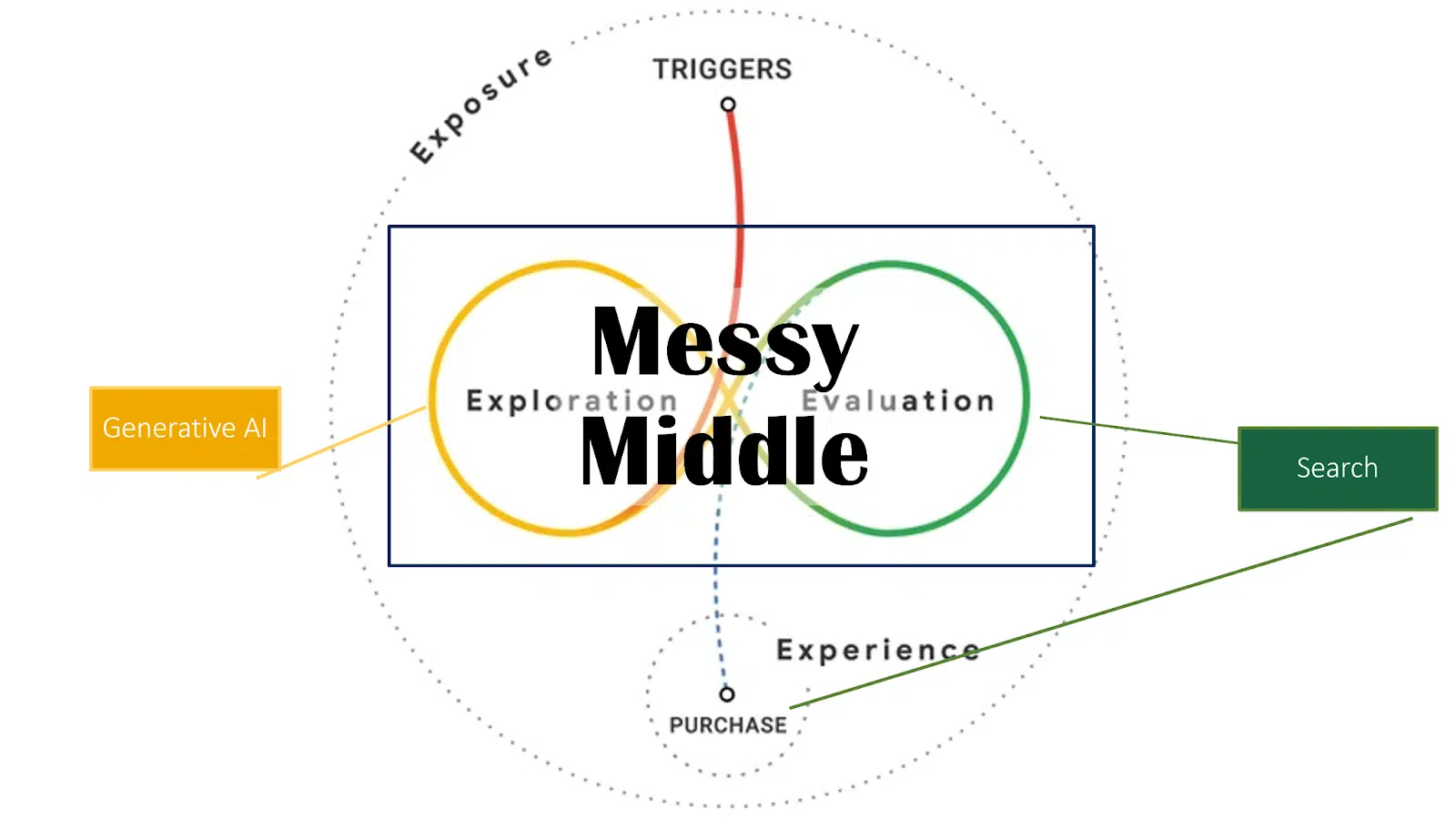
An AI snippet box was delivered in the Search Generative Experience for almost 26% of ecommerce-related search queries, per an SE Ranking study. Ecommerce was one of the five sectors most affected by SGE.
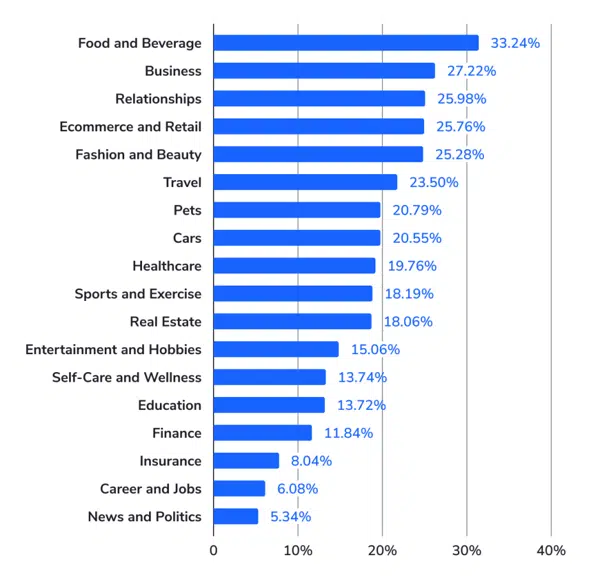
In many cases, the classic search results were replaced by SGE below the fold. A prominent component of product-related search queries are displayed from the Google Shopping Graph.

In the ecommerce sector, almost 80% of the sources ranked for the SGE were not in the top 10 search results for the respective search query, per a ZipTie.dev study.
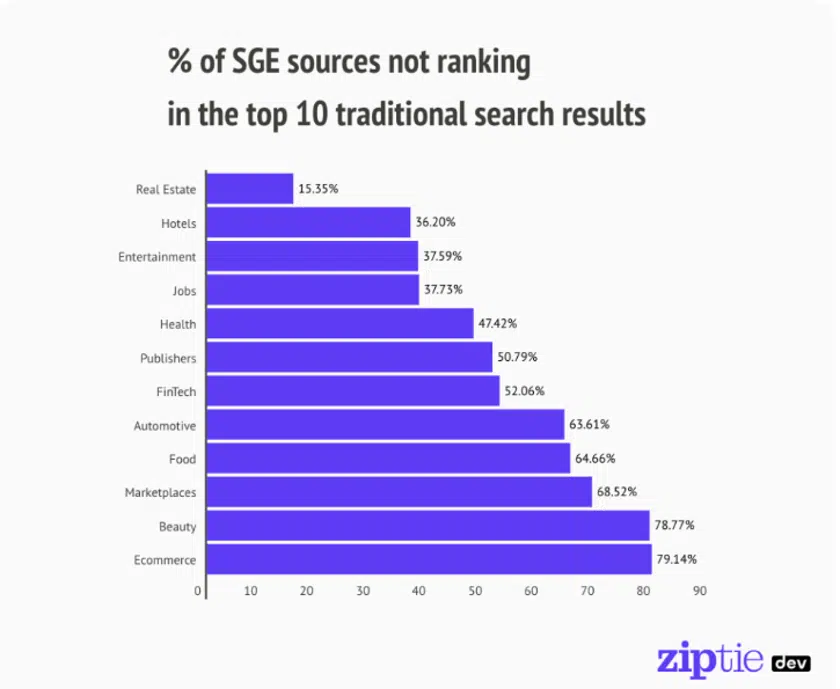
This means that we can only make limited progress with classic SEO. You have to start somewhere else and in my opinion that is the shopping graph.
Since 2012, the Knowledge Graph has been Google’s semantic database, in which the world’s knowledge about entities (nodes) and their relationships to one another (edges) is recorded and understood.
As a counterpart to the Knowledge Graph, Google built the Shopping Graph based on the same principle, focusing on product entities.
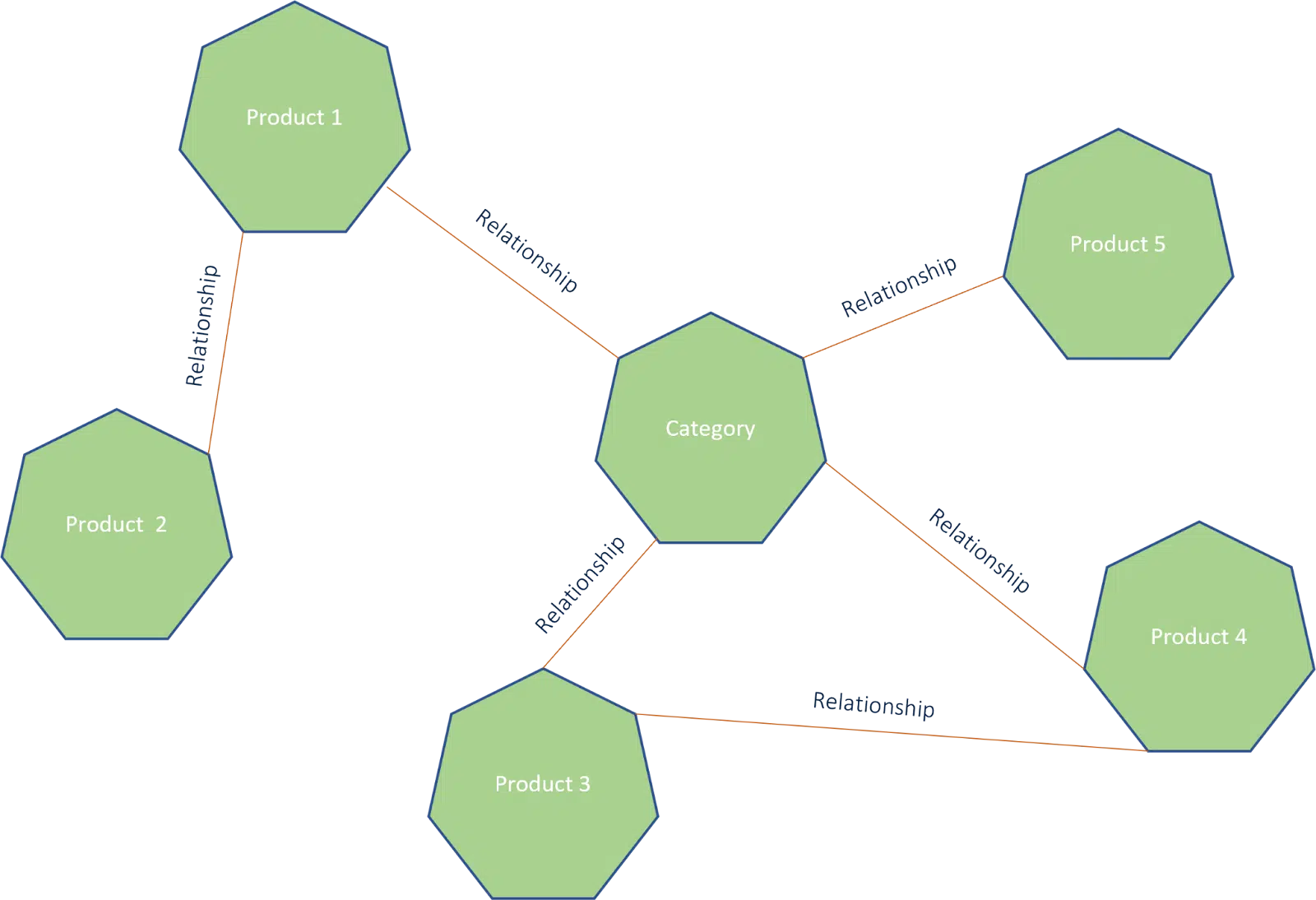
Google’s Shopping Graph is a massive, machine learning-powered database with billions of product listings, helping users find specific products.
- Google’s Shopping Graph is a real-time database of products and sellers powered by machine learning.
- With over 35 billion products, the Shopping Graph offers a huge selection of products and their details, such as availability, reviews, materials, colors and sizes.
- Users can search for products using specific criteria and the Shopping Graph scours billions of listings and relevant data across the web to find matching options.
- The Shopping Graph enables various shopping functions such as “Shop the Look” for styling ideas and “Buying Guide” for purchase recommendations by synthesizing information from various sources on the web.
- The Shopping Graph allows users to find inspiring products on Google and narrow down their options based on current shopping information.
To find clues for shopping graph optimization, you first have to consider where you should optimize. To do this, you need to know which data sources the information in the shopping graph is based on.
Google states that the information in the Shopping Graph comes from the following sources:
- YouTube-videos.
- Manufacturer websites.
- Online shops and product detail pages (PDPs).
- Google Merchant Center.
- Google Manufacturer Center.
- Product testing.
- Product reviews.
These sources are structured and unstructured. Structured info helps Google train their machine learning as manually labeled data with the goal of better understanding unstructured content via natural language processing.
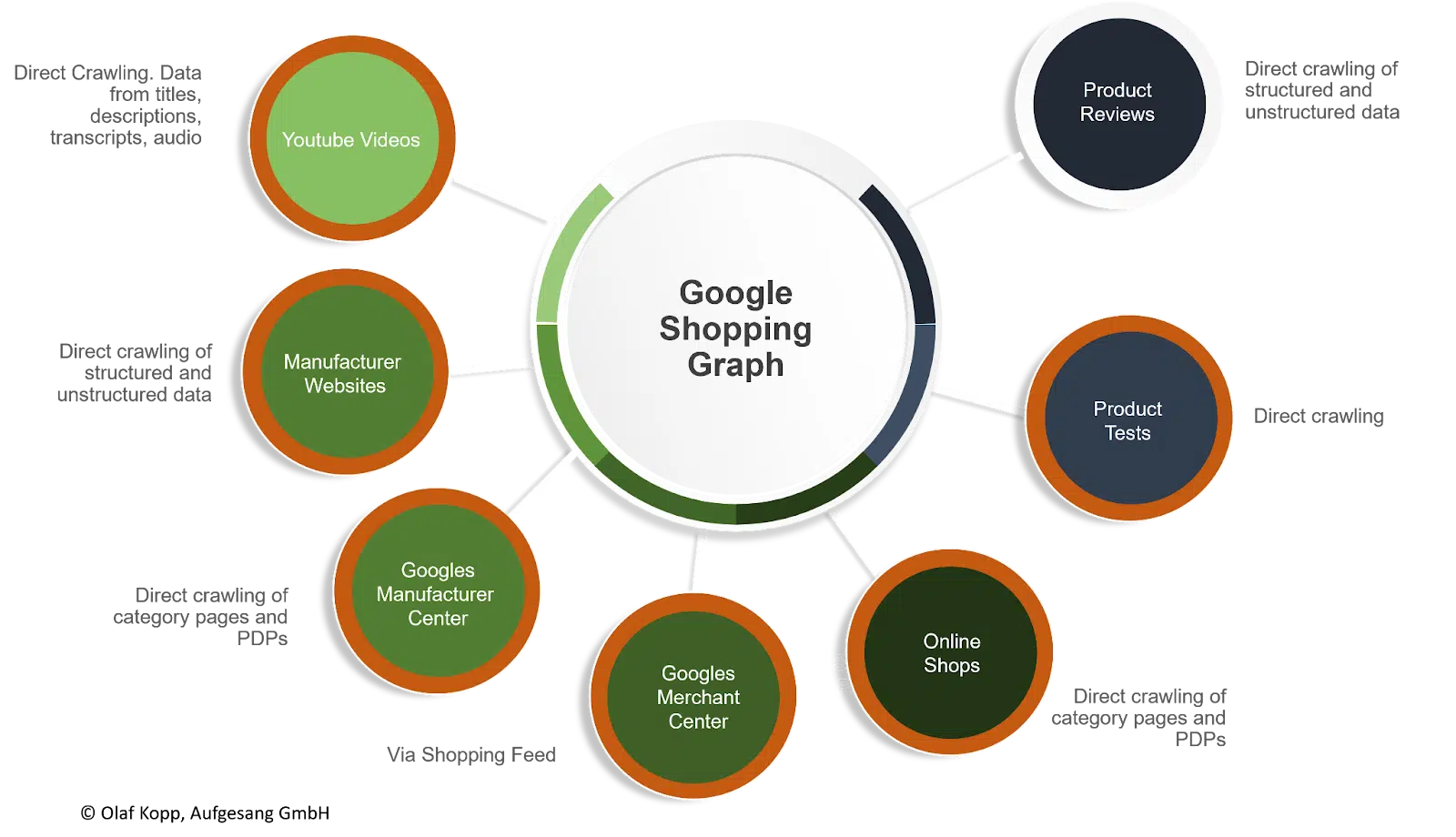
The Google Manufacturer Center is a tool provided by Google that allows manufacturers to feed detailed product information directly into Google’s shopping database.
This information can then be displayed in various Google services, including Google Shopping and Google Search results.
The Manufacturer Center aims to improve product display and increase the visibility and accuracy of product information, which can ultimately lead to a better online shopping experience for consumers.
Get the daily newsletter search marketers rely on.
RAG stands for “retrieval-augmented generation” and is a technique in artificial intelligence, specifically in natural language processing. RAG combines two main components: information retrieval and generative language ******.
The goal of RAG is to improve the quality and relevance of answers generated by language ****** by retrieving additional information from an external data source and using it to generate answers.
How RAG works:
- Retrieval: First, a search query is made to an external database to find relevant information. This can be a collection of texts, databases, graph databases or any other form of unstructured and structured data.
- Augmentation: The retrieved information is then fed as context into the generative model, which then generates a detailed and informed response.
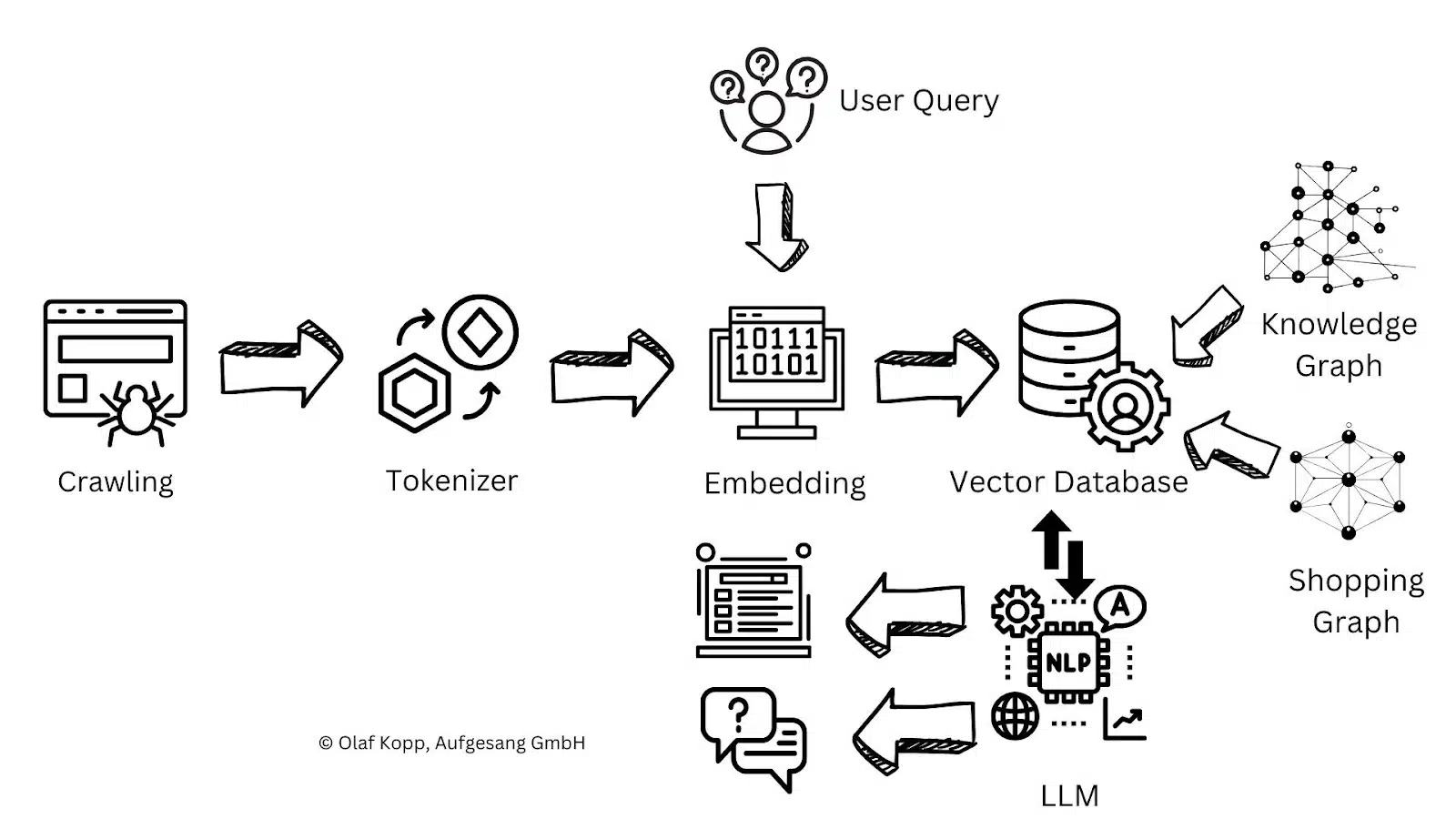
The Google Shopping Graph can be a valuable source of information for RAG-based systems, especially in ecommerce and online shopping applications, such as search engines.
Here are some possible roles of the Shopping Graph in a RAG system:
- Improving product research: For a product-specific query, a RAG system could pull relevant information from the Shopping Graph to generate more precise and contextually appropriate responses. For example, it could integrate specific product recommendations, availability data or pricing information.
- Personalized recommendations: The Shopping Graph could be used to generate personalized shopping recommendations based on the user’s specific interests and behavior stored in the Shopping Graph data.
- Supporting interactive queries: In an interactive chatbot scenario, the Shopping Graph could help respond to follow-up questions by providing additional product details or alternative suggestions based on the initial recommendations.
- Ratings and reviews integration: The Shopping Graph could also be used to include ratings and reviews in the generated responses, increasing the recommendations’ quality and usefulness.
Overall, the Shopping Graph can be key in optimizing RAG-based systems such as Google’s AI Overviews through its rich and structured information about products and their relationships.
Dig deeper: How Search Generative Experience works and why retrieval-augmented generation is our future
Large language ****** (LLMs) learn based on the frequency of co-occurrences that occur or, in the context of ecommerce, from co-mentions of attributes with the respective product.
The frequency of the attributes requested in prompts and search queries determines which attributes are important for a product entity.
Future product research will be more interactive and contextual. Prompts allow requests to be given many more levels of context. Here is an example of a product-related prompt.
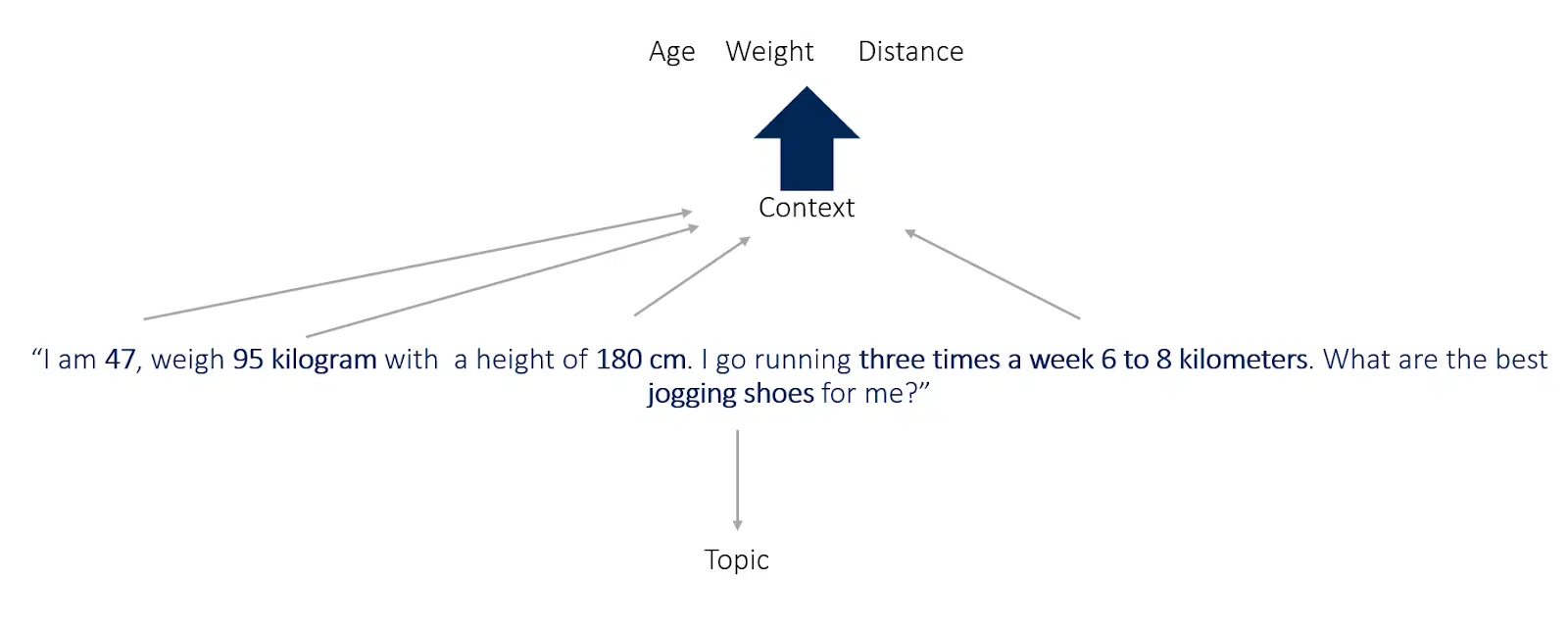
The topic of the prompt is jogging shoes or running shoes. Contexts are:
- Age: Middle-aged
- Weight: Overweight (weight to height)
- Distance: Medium distance
- Size: Medium-sized person
- Frequency: Frequent
With this prompt, the different AI systems give us different product recommendations:
ChatGPT suggests specific running shoe ****** and translates the context from the prompt into corresponding attribute types:
- Durability
- Foot Type
- Gait
- Fit
- Comfort
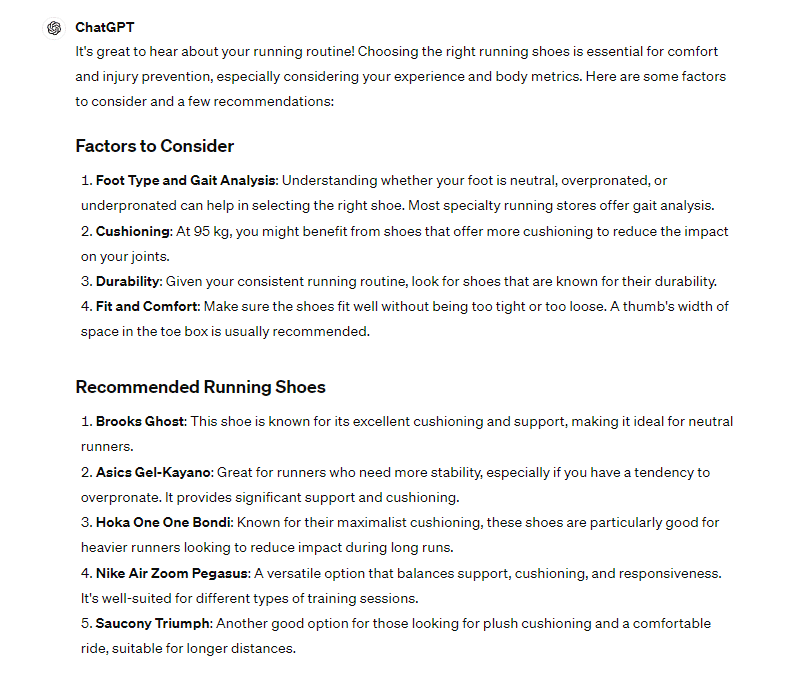
Google’s Gemini only suggests running shoe brands on the first attempt and translates the prompt into the following attributes:
- Cushioning
- Support
- Stability
- Running style
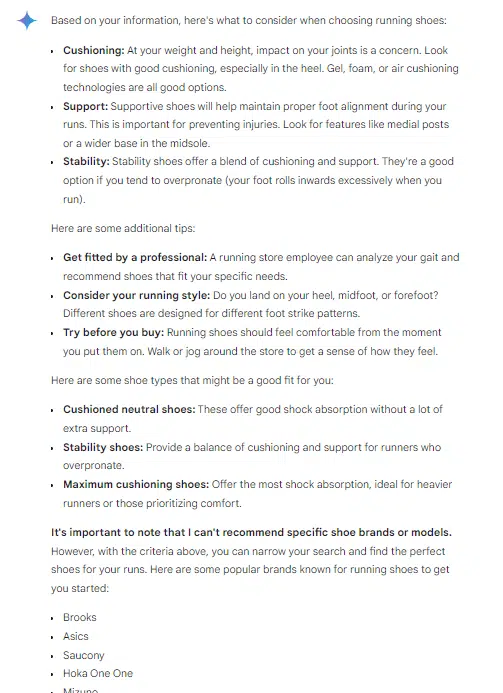
If you ask Gemini to specify the shoe ******, the following shoe ****** (with images) are suggested.
- Brooks Ghost 15
- Saucony Kinvara 14
- Asics Kayano 29
- Brooks Adrenaline GTS 23
- Hoka One One Bondi 8
- Saucony Triumph 20

The recommendations from both LLMs are similar. ChatGPT also suggested Brooks Ghost, Asics Kayano, Hoka One One Bondi and Saucony Triumph.
My tests have shown that this is not always the case and that product recommendations can vary. This may be related to the different training data.
So, why are these products suggested by the LLMs and not others?
These products often seem to be mentioned in the vicinity of the attributes translated in the respective LLM.
When optimizing for the shopping graph, you should mention the relevant attributes in the data sources mentioned above, if possible.
Here, we used our custom GPT for text analysis via natural language processing to analyze the manufacturer’s description of the Asics running shoes for the Asics Kayano model series.

The following attributes could be extracted from the manufacturer text:
- Age/longevity: The shoe has been ASICS’ pinnacle running shoe for the last 27 years.
- Popularity: It is described as one of the world’s most loved collections of running shoes.
- Design purpose: Specifically designed for long-distance running.
- Technological innovations: Receives the latest ASICS technology innovations with each model.
- Stability: Designed for stability with support and durability in mind.
- Gender-specific cushioning: Features cushioning that is specific to each gender.
- Comfort: Provides maximum comfort with a springy, supportive sole to prevent pain and rubbing during long runs.
- Improvement: The collection is constantly being improved and enhanced.
- Responsiveness: Described as springy, most responsive and easy to control.
I examined this YouTube video for sub-entities and attributes using the Chrome extension Harpa.ai in combination with Gemini.
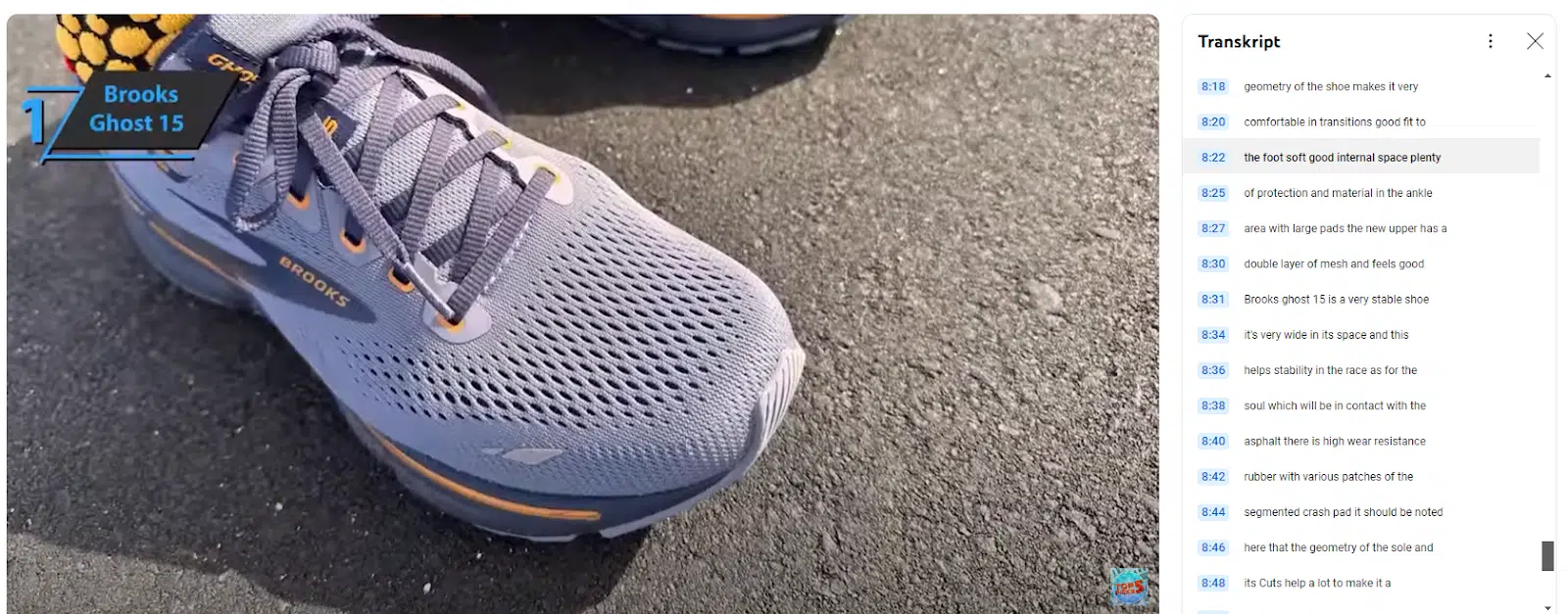
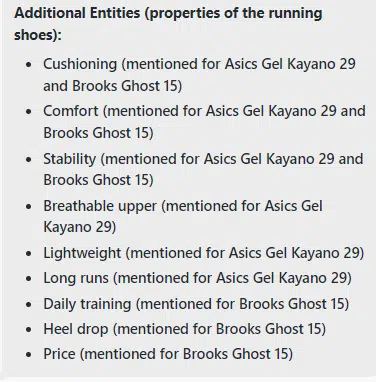
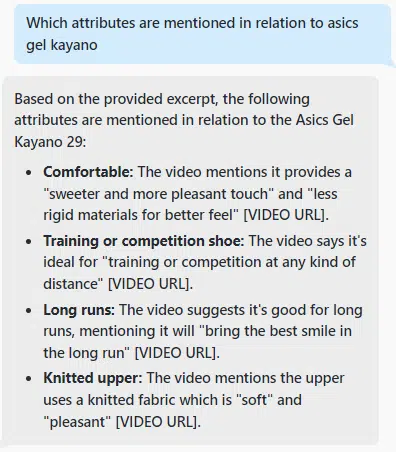
The running shoe model is associated with the attributes “comfortable,” “training or competition shoe,” “long distance” and “specific upper material.”
In this way, all possible data sources can be examined. The more the attributes associated with the respective product resemble the context specified in the prompt and the attributes derived from the LLM, the more likely the products will be mentioned in a response from the generative AI.
What can ecommerce brands learn from this?
The way ecommerce SEO has worked so far will evolve due to changes in research behavior brought about by generative AI such as AI Overviews, ChatGPT and Copilot.
Shop category pages will attract less and less organic traffic and users will increasingly be introduced to products through generative AI or LLMs. The extent to which this shift will occur is unclear.
However, we as SEOs should prepare for this hybrid approach to information search and use large language model optimization in ecommerce to avoid losing visibility.
The semantic heart of this is the shopping graph as a product entity database. The Shopping Graph, a semantic, machine learning-based database, contains extensive product information and is central in connecting users with products through specific search criteria.
Optimization options for the shopping graph arise from various data sources such as YouTube videos, manufacturer websites and online shops or the design of the product detail pages and the shopping feeds.
Key takeaways on the future of ecommerce SEO
- Focus on shopping graph optimization: SEO strategies should focus on optimization within the Google Shopping Graph as it becomes increasingly important, especially through its integration with AI Overviews.
- Adapt to generative AI: Since generative AI changes search behavior by making research faster and more interactive, ecommerce SEO efforts should be focused on being present in the shopping graph data sources and emphasizing the relevant attributes.
- Consider new search behaviors: With the increase in AI-powered tools, classic search results are increasingly being clicked on less. SEO and marketing strategies must adapt to requiring fewer touchpoints to reach and convince users.
- Optimize based on data sources: Effective shopping graph optimization strategies should focus on improving the context and relevance of products in the primary data sources for the Shopping Graph, such as YouTube, manufacturer websites and product reviews.
- Identify and understand user and product-relevant attributes: Long-tail analysis of search queries and prompts is becoming increasingly important.
- Refine your knowledge of entities and semantic search: A semantic understanding of entity-based information retrieval systems and a technological understanding of LLMs will be one of the foundations of SEO in the future.
- Think beyond keywords: SEOs must think in terms of concepts, entities, attributes and relationships. The time of keywords as a central focus is coming to an end.
Opinions expressed in this article are those of the guest author and not necessarily Search Engine Land. Staff authors are listed here.
Source link : Searchengineland.com